Heart Rate Variability
What is Heart Rate Variability (HRV)?
Heart rate variability (HRV) is the change in time from one R peak of a QRS cycle to the next throughout the duration of a recording (as seen in the figure below). It is closely associated with the autonomic regulation of cardiac functioning and can act as a measure of sympathetic and parasympathetic interactions (Thayer 2006; Shaffer et al. 2014). Individuals with lower HRV are thought to have less adaptability to stress, and low HRV has been linked to greater physiological and psychological health problems.
There are numerous ways to measure HRV, which has led to a wide variety of methodological differences between studies. Two articles (Laborde et al. 2017; Malik et al. 1996) have set forth suggestions for universal methods and measures to be implemented across studies. However, not all of the suggestions in these articles are followed or possible based on study design. Thus, it is critical to compare methods and agreement between studies before comparing results.
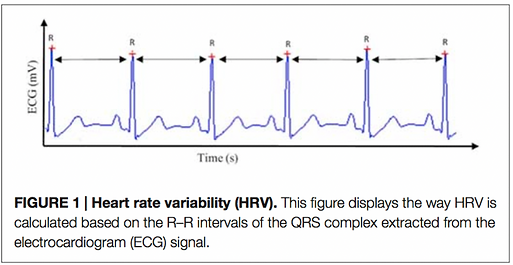.png)
Laborde S, Mosley E, Thayer JF. 2017. Heart rate variability and cardiac vagal tone in psychophysiological research – Recommendations for experiment planning, data analysis, and data reporting. Front Psychol. 8:213. doi:10.3389/fpsyg.2017.00213.
Data collection
Device
The standard for measuring HRV is ECG recording, which measures HRV from the raw heart rate waveform. However, there are several other devices, such as finger monitors and chest straps, that record inter-beat intervals (IBI) and can also be used to analyze HRV. Unlike ECG, devices that record IBI only measure the interval times between R peaks, and thus are more difficult to inspect so as to ensure quality data (Laborde et al. 2017). Thus, it is important to assess the reliability of a device in comparison to ECG recording before assessing data.
Lead configuration
There are many different lead configurations for measuring ECG. One way to collect ECG data is through a lead II configuration, where one electrode is placed just under the right collarbone, and the other electrode is placed on the left side of the body on the rib cage.
Sampling rate
There is no set sampling rate for collecting ECG data, however, it has been proposed that ECG data should be sampled between 250-1000 Hz (Malik et al. 1996). One study tested this suggestion, by examining lead II ECG recordings sampled at 1000 Hz, and down-sampling these recordings to 19 different frequencies (Ellis et al. 2015). This study found that frequencies down to 125 Hz without interpolation yielded consistent results compared to 1000 Hz, confirming the suggestions of Malik et al. (1996). Another study that examined emergency room patients, rather than healthy patients, found similar results after down-sampling a 1000 Hz ECG recording to 500, 250, 100, and 50 Hz (Kwon et al. 2018). These two studies demonstrate that acceptable ECG sampling frequencies can range from 250 Hz (even as low as 100 Hz in some cases) to 1000 Hz and still yield comparable results, thus further reinforcing the suggestions provided in Malik et al. (1996).
Analysis
Artifact correction
Artifact correction is a critical part of HRV analysis. Artifacts (missed, abnormal, or added beats) can significantly alter the results of an analysis, and thus need to be corrected (Berntson and Stowell 1998). Artifact correction can be performed manually, however, there is also a function in the Kubios Premium software that allows for automatic artifact correction (Lipponen and Tarvainen 2019). This function detects artifacts through a decision-based algorithm that works in conjunction with Kubios’s RR interval detection algorithm.
Analysis parameters
The three major domains of HRV analysis are time-domain, frequency-domain, and non-linear analysis. Each method of analysis has different parameters that act as measures of various physiological functions (Laborde et al. 2017).
.png)
Laborde S, Mosley E, Thayer JF. 2017. Heart rate variability and cardiac vagal tone in psychophysiological research – Recommendations for experiment planning, data analysis, and data reporting. Front Psychol. 8:213. doi:10.3389/fpsyg.2017.00213.
Fast Fourier transform (FFT)
Of the three HRV analysis methods, frequency-domain parameters are common measures reported in the literature. There are two main methods for a frequency analysis; fast Fourier transformation (FFT) and autoregressive modeling (AR) (Laborde et al. 2017). Each of these methods has certain advantages and disadvantages, and which method is used will vary from study to study.
An FFT analysis measures HRV through estimating power from power spectral density (PSD) calculations (Malik et al. 1996). Data is windowed, which helps to reduce spectral leakage while preserving spectrum resolution (Badilini and Blanche 1996). One consideration a researcher must take into account for an FFT analysis is what type of window and window settings will be used. For a five-minute sample, Kubios recommends a Hanning window with a window length of 150 samples, an overlap of 50%, and an FFT width of 512 samples (Tarvainen MP, email correspondence, 2020). Additionally, when data is windowed, the power of the spectrum becomes mismatched with the variance of the original signal (Badilini and Blanche 1996). In order to adjust for this, a scale factor is typically applied. For Kubios, the scale factor is found as equation 1 in Badilini and Blanche (1996), while for AcqKnowledge there is no scale factor.
Log transformation
Since HRV measures typically result in a non-normal distribution, it is common in the literature to transform the data by taking the natural log (LN) prior to statistical analysis (Laborde et al. 2017). This can be verified by creating histograms of the distribution before and after transformation to verify that data has been properly treated.
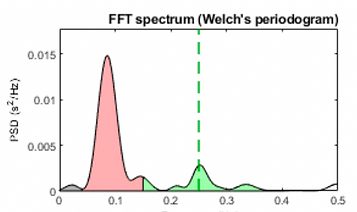

The frequency band spectrums calculated through FFT and AR analyses in Kubios (grey = VLF, red = LF, green = HF)
.png)
RMSSD distribution prior to LN transformation
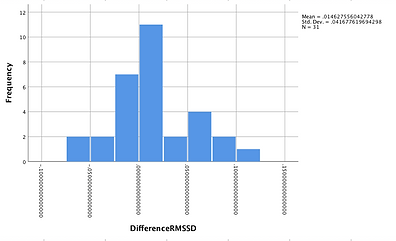.png)
RMSSD distribution after LN transformation
Kubios methods
A standard software for HRV analysis is Kubios (Tarvainen et al. 2014), which allows for automated analysis of HRV data. The methods Kubios uses for HRV analysis can be found on their website (About HRV… 2020). Additionally, further information is provided in this statement from Kubios support (Tarvainen MP, email correspondence, 2020):
“Before both spectrum estimates the RR data is interpolated (by default at 4 Hz) using cubic spline, to have equidistantly sampled data.
FFT spectrum estimate:
-
We use Welch's periodogram approach, where the spectrum is computed by averaging spectra from overlapping windows. The window width and overlap can be adjusted from preferences. Typically, I recommend that you should have at least couple of windows to average, e.g. in case of 5-min analysis sample, you could set the window width=150 secs, overlap=50%; to have three overlapping windows. In addition, you can adjust the points in frequency domain Nfft. if Nfft is higher than points within a windowed data, then zero padding is applied to do spectral interpolation.
-
The spectral resolution of FFT spectrum depends on the window width, the longer the window the better the frequency resolution. For a 150 sec window, the frequency resolution is roughly 1/150s ~ 0.007 Hz
-
Spectral power is scaled with the sampling frequency, length of data and window function (we use Hanning window inside the Welch's periodogram). The scaling is done according to Parseval's theorem, meaning that total power of spectrum is equal to variance of the data.
AR spectrum estimate:
-
You can adjust the AR model order in this approach, which also defines the frequency resolution of the spectra. In theory the AR method has better frequency resolution due to implicit extrapolation of the data, but in practical application this may be insignificant.
-
The AR model coefficients are solved using a forward-backward least squares method and the AR spectrum is scaled according to Parseval's theorem (model residual variance and sampling frequency are required for this).”
References
About HRV - Kubios HRV. 2020. Kuopio, Finland: Kubios Oy; [accessed 2020 June 22]. https://www.kubios.com/about-hrv/#top
Badilini F, Blanche P. 1996. Hrv spectral analysis by the averaged periodogram: Does the total power of the spectrum really match with the variance of the tachogram? Annals of Noninvasive Electrocardiology. 1(4):423–429. doi:10.1111/j.1542-474X.1996.tb00300.x.
Berntson GG, Stowell JR. 1998. ECG artifacts and heart period variability: Don’t miss a beat! Psychophysiol. 35(1):127–132. doi:10.1111/1469-8986.3510127.
Ellis RJ, Zhu B, Koenig J, Thayer JF, Wang Y. 2015. A careful look at ECG sampling frequency and R-peak interpolation on short-term measures of heart rate variability. Physiol Meas. 36(9):1827–1852. doi:10.1088/0967-3334/36/9/1827.
Kwon O, Jeong J, Kim HB, Kwon IH, Park SY, Kim JE, Choi Y. 2018. Electrocardiogram sampling frequency range acceptable for heart rate variability analysis. Healthc Inform Res. 24(3):198–206. doi:10.4258/hir.2018.24.3.198.
Laborde S, Mosley E, Thayer JF. 2017. Heart rate variability and cardiac vagal tone in psychophysiological research – Recommendations for experiment planning, data analysis, and data reporting. Front Psychol. 8:213. doi:10.3389/fpsyg.2017.00213.
Lipponen JA, Tarvainen MP. 2019. A robust algorithm for heart rate variability time series artefact correction using novel beat classification. J of Med Engr & Tech. 43(3):173–181. doi:10.1080/03091902.2019.1640306.
Malik M, Bigger JT, Camm AJ, Kleiger RE, Malliani A, Moss AJ, Schwartz PJ. 1996. Heart rate variability: Standards of measurement, physiological interpretation, and clinical use. Eur Heart J. 17(3):354–381. doi:10.1093/oxfordjournals.eurheartj.a014868.
Tarvainen MP, Niskanen J-P, Lipponen JA, Ranta-aho PO, Karjalainen PA. 2014. Kubios HRV – Heart rate variability analysis software. Comp Meth and Pgrm in Biomed. 113(1):210–220. doi:10.1016/j.cmpb.2013.07.024.